Personalizing Assistance for Exoskeletons Matches User Need
Personalizing Assistance for Exoskeletons Matches User Need
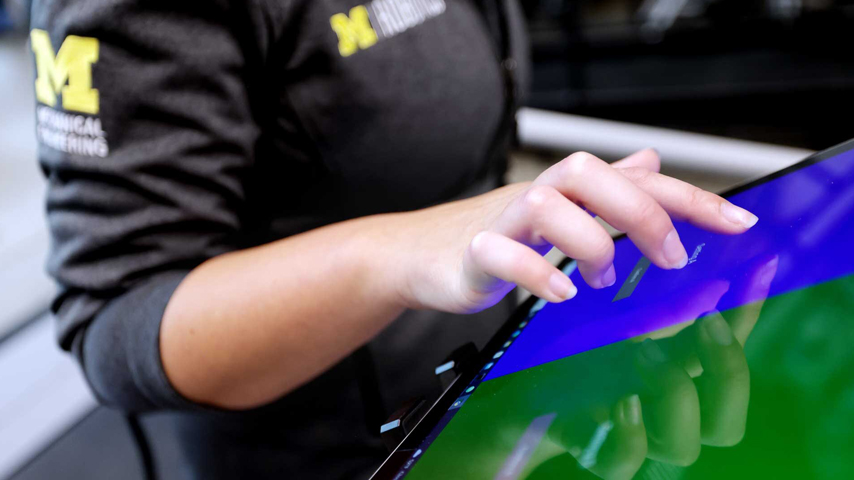
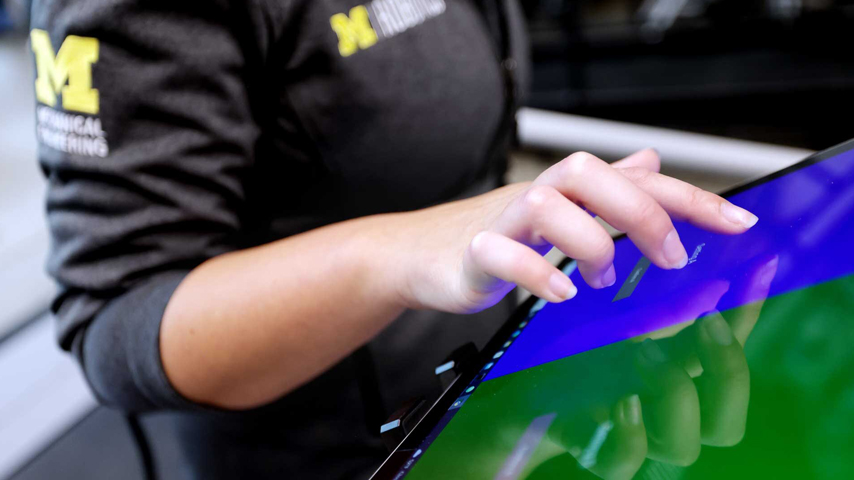
To operate the instructions, two assistance profiles are selected based on previous data. The user chooses one, and it presents another for comparison.
Exoskeletons, or wearable structures that can assist movement, have the potential to not only augment human strength and endurance but also help those with mobility issues remain independent longer. As research groups across the globe work to enhance these mechanical scaffolds using a variety of different sensors and robotic elements, there is one aspect that has been overlooked, said Elliott Rouse, an associate professor of robotics and mechanical engineering at the University of Michigan: How to adjust the assistance exoskeletons provide to match user preferences.
"Ankle exoskeletons work by applying some type of assistance as a person walks forward, but each person may want the shape of that assistance to be a bit different,” he explained. “We’re trying to create instructions to assistance for these devices and find a way to make those instructions cooperative, helpful, and enjoyable.”
Traditionally, such preferences are selected in one of two ways. Expert-tuned is where a researcher or clinician makes adjustments to the control strategy based on observations in things like biomechanical trajectories. Human-in-the-loop optimization is where control parameters change based on user-specific parameters like metabolic rate.
The former takes control out of the hands of the wearer, who may not be able to articulate the kind of support they want. As for the latter, since most individuals have little conscious awareness of how many calories they may be burning as they walk, it may not support provide the kind of assistance that support user preferences either, Rouse said.
"Right now, metabolic rate is the gold standard for exoskeleton assessment. But if we’re optimizing for a metric that people can’t perceive, they probably won’t sense that as beneficial,” he said. “Our previous work shows that people have very strong preferences about how they would like the exoskeleton to help them. Our goal was to find a way to curate the assistance profile in the way they want it.”
To that end, Rouse and colleagues developed a machine-learning algorithm that offers users pairs of assistance profiles to control exoskeleton settings including peak torque, timing, and how the wearable ramps up and reduces the assistance. After looking at a series of user selections between pairs of profiles, the algorithm makes a suggestion for a profile that may match the users’ preferences better. Rouse likened the approach to the thumbs up/thumbs down interface seen in online radio apps like Spotify and Pandora.
Become a Member: How to Join ASME
"I use those radio stations. They receive input from the user based on their experience and then, with a thumbs up or thumbs down, they can offer suggestions about other stations that people may like,” he said. “Since people have such clear thoughts on what they want from an exoskeleton, we could use those preferences to help them get to an optimized profile.”
To test whether the algorithm could provide proper assistance curation, the research team recruited 14 participants to wear ankle exoskeletons as they walked at a steady 2.3-mile-per-hour pace on a treadmill. A touchscreen controller would offer them choices between assistance profiles. After making 50 choices―where most participants were selecting the same profile by the 45th decision―the algorithm helped shape an optimized profile based on their previous selections.
To see whether the algorithm really helped users select the best assistance profile, the experiment then asked participants to choose between it and 10 other randomly generated profiles. Nine out of 10 times, the users kept the settings curated by the algorithm. Rouse said they learned some important lessons but were buoyed by the fact that the algorithm got the right profile 90 percent of the time.
More for You: Exoskeleton Boot Improves Walking Speed
"We learned that people’s preferences are really invariant around timing. Almost everyone wants the same timing, and it’s only separated by a few milliseconds,” he said. “Given that preferences can change, and that people aren’t always so good at articulating what their preferences are, I think we showed this approach is a good one.”
Even with 90 percent accuracy, however, Rouse said the team still has some work to do to “streamline” the system. Rouse is motivated to create a viable system that can be translated for real-world use.
Right now, this works on a tablet; it’s a PC tablet mounted to the treadmill. For our goals, we’d probably want the system to live on a watch or a smart phone. Finding a way to improve technological access, so it can happen unobtrusively, would be a good thing,” he said.
"But we also have some improvements to make with the algorithm. As it is, the algorithm does not learn. So, if your preferences change, it wouldn’t necessarily track that your preference had changed. It would try to find a profile that was somewhere in the middle of your past and current preference. We need to work on that so we can give it more robustness. Then we can look at how people could use this to optimize their assistance profiles as needed.”
Kayt Sukel is a science and technology writer in Spring, Texas.
"Ankle exoskeletons work by applying some type of assistance as a person walks forward, but each person may want the shape of that assistance to be a bit different,” he explained. “We’re trying to create instructions to assistance for these devices and find a way to make those instructions cooperative, helpful, and enjoyable.”
Traditionally, such preferences are selected in one of two ways. Expert-tuned is where a researcher or clinician makes adjustments to the control strategy based on observations in things like biomechanical trajectories. Human-in-the-loop optimization is where control parameters change based on user-specific parameters like metabolic rate.
The former takes control out of the hands of the wearer, who may not be able to articulate the kind of support they want. As for the latter, since most individuals have little conscious awareness of how many calories they may be burning as they walk, it may not support provide the kind of assistance that support user preferences either, Rouse said.
"Right now, metabolic rate is the gold standard for exoskeleton assessment. But if we’re optimizing for a metric that people can’t perceive, they probably won’t sense that as beneficial,” he said. “Our previous work shows that people have very strong preferences about how they would like the exoskeleton to help them. Our goal was to find a way to curate the assistance profile in the way they want it.”
To that end, Rouse and colleagues developed a machine-learning algorithm that offers users pairs of assistance profiles to control exoskeleton settings including peak torque, timing, and how the wearable ramps up and reduces the assistance. After looking at a series of user selections between pairs of profiles, the algorithm makes a suggestion for a profile that may match the users’ preferences better. Rouse likened the approach to the thumbs up/thumbs down interface seen in online radio apps like Spotify and Pandora.
Become a Member: How to Join ASME
"I use those radio stations. They receive input from the user based on their experience and then, with a thumbs up or thumbs down, they can offer suggestions about other stations that people may like,” he said. “Since people have such clear thoughts on what they want from an exoskeleton, we could use those preferences to help them get to an optimized profile.”
To test whether the algorithm could provide proper assistance curation, the research team recruited 14 participants to wear ankle exoskeletons as they walked at a steady 2.3-mile-per-hour pace on a treadmill. A touchscreen controller would offer them choices between assistance profiles. After making 50 choices―where most participants were selecting the same profile by the 45th decision―the algorithm helped shape an optimized profile based on their previous selections.
To see whether the algorithm really helped users select the best assistance profile, the experiment then asked participants to choose between it and 10 other randomly generated profiles. Nine out of 10 times, the users kept the settings curated by the algorithm. Rouse said they learned some important lessons but were buoyed by the fact that the algorithm got the right profile 90 percent of the time.
More for You: Exoskeleton Boot Improves Walking Speed
"We learned that people’s preferences are really invariant around timing. Almost everyone wants the same timing, and it’s only separated by a few milliseconds,” he said. “Given that preferences can change, and that people aren’t always so good at articulating what their preferences are, I think we showed this approach is a good one.”
Even with 90 percent accuracy, however, Rouse said the team still has some work to do to “streamline” the system. Rouse is motivated to create a viable system that can be translated for real-world use.
Right now, this works on a tablet; it’s a PC tablet mounted to the treadmill. For our goals, we’d probably want the system to live on a watch or a smart phone. Finding a way to improve technological access, so it can happen unobtrusively, would be a good thing,” he said.
"But we also have some improvements to make with the algorithm. As it is, the algorithm does not learn. So, if your preferences change, it wouldn’t necessarily track that your preference had changed. It would try to find a profile that was somewhere in the middle of your past and current preference. We need to work on that so we can give it more robustness. Then we can look at how people could use this to optimize their assistance profiles as needed.”
Kayt Sukel is a science and technology writer in Spring, Texas.
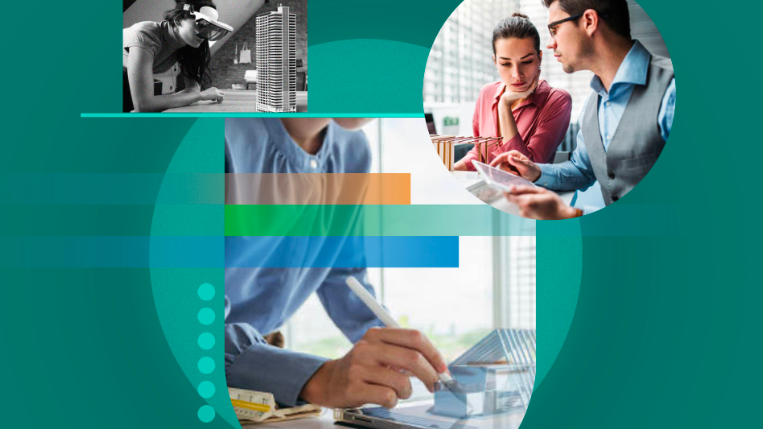
Pulse of the Profession: CAD in 2030
CAD technologies and their applications are rising in tandem with trends in Industry 4.0.