Better Flaw Detection During Powder Bed Fusion
Better Flaw Detection During Powder Bed Fusion
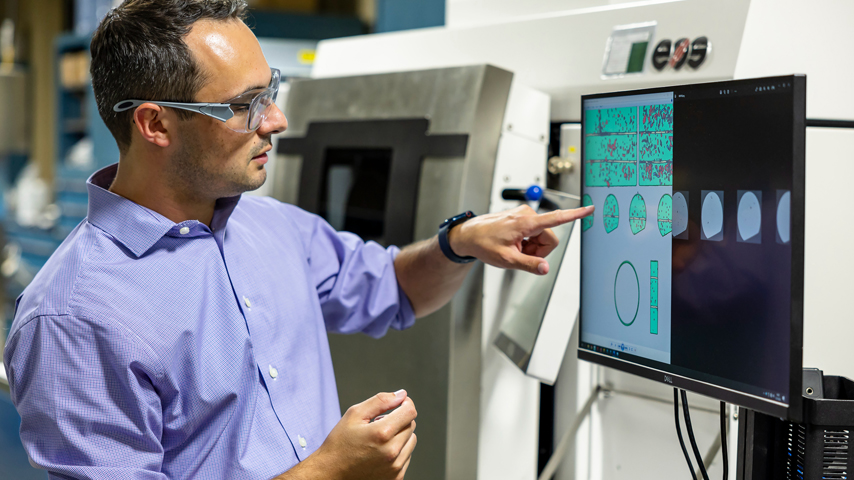
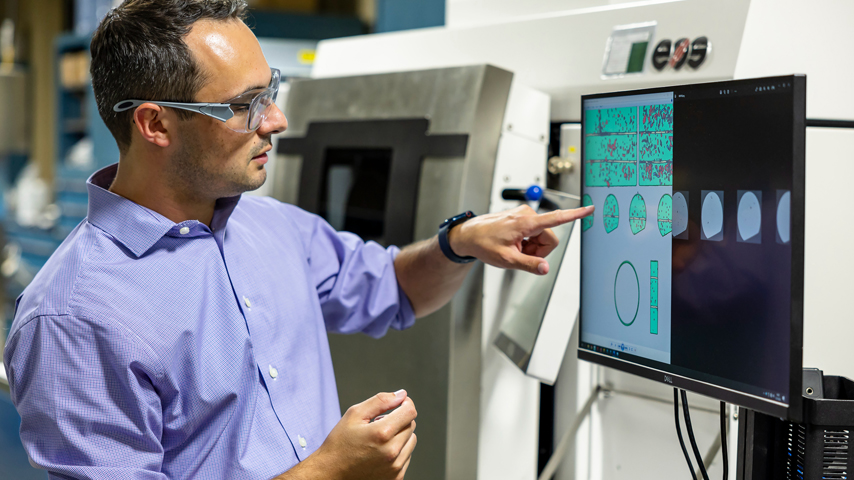
Researchers have developed a new framework for quantifying the performance of flaw detections using in-situ monitoring and machine learning for powder bed fusion 3D printing.
Powder bed fusion, an additive manufacturing method, is an unpredictable and stochastic process as it involves turbulently melting metal powder in an argon environment to fuse it in place. In certain instances, a portion of this pool of melted metal is ejected and propelled around the build surface as spatter particles.
Researchers at Oak Ridge National Laboratory and RTX Technology Research Center have developed a new method for analyzing in situ monitoring data collected during powder bed fusion 3D printing, which will allow engineers to incorporate the right safety factors into their designs.
By studying the spatter particles during the printing process, the research team developed “a framework to detect a particular type of stochastic flaw with a quantifiable level of confidence. This flaw probability could be given to design engineers to implement into future designs,” explained Zackary Snow, an associate staff scientist at Oak Ridge National Laboratory in Tennessee.
More on Advanced Manufacturing: 3D-Printed Ceramic Turbine Parts Withstand High Temperatures
The in-situ monitoring setup includes a high resolution visible light camera with a spatial resolution ranging from 50 to 75 microns, depending on the printing configuration. A near infrared camera supplies information on the thermal behavior experienced during the welding process of each 2D layer. A machine learning tool with cameras identifies anomalies and defects. The operators can add observed anomalies back into the training database for the machine learned tool to recognize in future builds.
“If we can provide it with enough examples of different geometries, different types of failure modes, different materials, then we can give it enough examples that it can handle those situations it hasn't yet seen,” explained Snow.
Powder bed fusion divides the part geometry into a series of 2D layers. The printer spreads a thin layer of metal powder across the build plate, a laser traces the first layer of the 2D images, melts the metal powder, and fuses it to the build plate. Subsequently, the build plate descends, more powder is added, and the laser fuses the second layer. This repetition continues until all layers have been lasered, completing the part.
The research team constructed a build plate with different test specimens to assess in-situ monitoring of spatter particles during a range of powder bed fusion builds. “At the melt pool scale, there are a lot of complex physics going on at the same time. A lot of our research was trying to find the one rogue stochastic anomaly that could end up in a defect,” Snow said.
Become a Member: How to Join ASME
Typical metal powder sizes are 50 microns, but spatter particles can reach up to 1 mm in diameter. When the spatter particles land on a part of the build, they don’t melt properly because the larger diameter requires more time and heat to weld. This results in a lack of fusion, which can mean defects in the build. The power and speed of the laser drive the stochastic behavior—the faster the print and stronger the laser, the more chaotic the process becomes, resulting in higher risk of spatter particles.
An advantage of powder bed fusion is its ability to produce intricate geometry that other production methods—such as stamping, casting, machining, or forging—cannot achieve. For example, the design of the part could require an internal cooling channel, achievable through 3D printing methods. However, in this scenario the channel would be completely internal and not inspectable once the part is complete. The quality assurance team could not inspect the geometry, thickness of the channel walls, and cross-sectional area of the channel for non-destructive evaluation (NDE).
“We have an opportunity to, even with really complicated geometries, inspect the quality of the material in this volumetric way that hasn't been available to us before,” Snow said.
Manufacturing Blog: Integrating AI into Production Processes
Monitoring the part during printing allows for detection of flaws and anomalies early in the build process. This could enable a technician to either cancel the print if the defect was significant enough to fail inspection or flag the area for further review by an NDE team. For production runs with extended print times, up to 10 days in some cases, identifying flaws on day one of a print can save considerable time and money to cancel the print and start over.
Industrial applications for powder bed fusion might involve one or a fleet of machines printing the same geometry to manufacture tens, hundreds, or thousands of the same part each day. Similar machines may have specific differences related to the manufacturer of the machine, purchase date, and performance capabilities.
Detecting anomalies early, canceling defective prints, and integrating machine learning could improve quality across printing machines and production runs, ensuring consistent high-quality production in industrial applications.
Nicole Imeson is an engineer and writer in Calgary, Alberta.
Researchers at Oak Ridge National Laboratory and RTX Technology Research Center have developed a new method for analyzing in situ monitoring data collected during powder bed fusion 3D printing, which will allow engineers to incorporate the right safety factors into their designs.
By studying the spatter particles during the printing process, the research team developed “a framework to detect a particular type of stochastic flaw with a quantifiable level of confidence. This flaw probability could be given to design engineers to implement into future designs,” explained Zackary Snow, an associate staff scientist at Oak Ridge National Laboratory in Tennessee.
More on Advanced Manufacturing: 3D-Printed Ceramic Turbine Parts Withstand High Temperatures
The in-situ monitoring setup includes a high resolution visible light camera with a spatial resolution ranging from 50 to 75 microns, depending on the printing configuration. A near infrared camera supplies information on the thermal behavior experienced during the welding process of each 2D layer. A machine learning tool with cameras identifies anomalies and defects. The operators can add observed anomalies back into the training database for the machine learned tool to recognize in future builds.
“If we can provide it with enough examples of different geometries, different types of failure modes, different materials, then we can give it enough examples that it can handle those situations it hasn't yet seen,” explained Snow.
A stochastic process
Powder bed fusion divides the part geometry into a series of 2D layers. The printer spreads a thin layer of metal powder across the build plate, a laser traces the first layer of the 2D images, melts the metal powder, and fuses it to the build plate. Subsequently, the build plate descends, more powder is added, and the laser fuses the second layer. This repetition continues until all layers have been lasered, completing the part.
The research team constructed a build plate with different test specimens to assess in-situ monitoring of spatter particles during a range of powder bed fusion builds. “At the melt pool scale, there are a lot of complex physics going on at the same time. A lot of our research was trying to find the one rogue stochastic anomaly that could end up in a defect,” Snow said.
Become a Member: How to Join ASME
Typical metal powder sizes are 50 microns, but spatter particles can reach up to 1 mm in diameter. When the spatter particles land on a part of the build, they don’t melt properly because the larger diameter requires more time and heat to weld. This results in a lack of fusion, which can mean defects in the build. The power and speed of the laser drive the stochastic behavior—the faster the print and stronger the laser, the more chaotic the process becomes, resulting in higher risk of spatter particles.
Complex geometry
An advantage of powder bed fusion is its ability to produce intricate geometry that other production methods—such as stamping, casting, machining, or forging—cannot achieve. For example, the design of the part could require an internal cooling channel, achievable through 3D printing methods. However, in this scenario the channel would be completely internal and not inspectable once the part is complete. The quality assurance team could not inspect the geometry, thickness of the channel walls, and cross-sectional area of the channel for non-destructive evaluation (NDE).
“We have an opportunity to, even with really complicated geometries, inspect the quality of the material in this volumetric way that hasn't been available to us before,” Snow said.
Manufacturing Blog: Integrating AI into Production Processes
Monitoring the part during printing allows for detection of flaws and anomalies early in the build process. This could enable a technician to either cancel the print if the defect was significant enough to fail inspection or flag the area for further review by an NDE team. For production runs with extended print times, up to 10 days in some cases, identifying flaws on day one of a print can save considerable time and money to cancel the print and start over.
Industrial applications for powder bed fusion might involve one or a fleet of machines printing the same geometry to manufacture tens, hundreds, or thousands of the same part each day. Similar machines may have specific differences related to the manufacturer of the machine, purchase date, and performance capabilities.
Detecting anomalies early, canceling defective prints, and integrating machine learning could improve quality across printing machines and production runs, ensuring consistent high-quality production in industrial applications.
Nicole Imeson is an engineer and writer in Calgary, Alberta.